Improving Health Outcomes Through Big Data with Theresa Walunas, PhD
In recent years, Feinberg has launched several initiatives to augment human expertise with computational methods and advance the science of human health. Theresa Walunas, PhD, explains how she is using big data from electronic medical records in a variety of projects at Feinberg, from improving quality of care to identifying patients who could develop debilitating autoimmune diseases.
Recorded on May 17, 2022
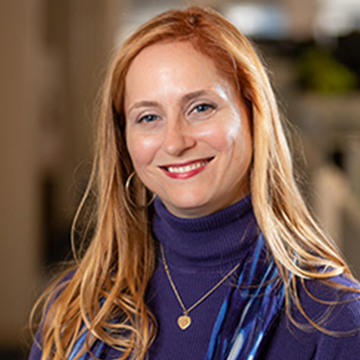
“What we're trying to do is not have the machine think for us, but trying to have the machine help us weed through the data and identify the things that are important so that our clinical care team members can make really good decisions about how to improve care and make sure patients at Northwestern Medicine get the best care possible.” — Theresa L. Walunas, PhD
About The Speaker
- Associate director of IPHAM’s Center for Health Information Partnerships
- Assistant Professor of Medicine in the Division of General Internal Medicine
- Assistant Professor in Microbiology-Immunology
- Assistant Professor of Preventive Medicine in the Division of Health and Biomedical Informatics
- Member of Northwestern University Clinical and Translational Sciences Institute
- Member of Robert H. Lurie Comprehensive Cancer Center
Episode Notes
In this episode, Walunas talks about several projects she has worked on and how the field of machine learning in medicine has grown in the last decade.
Topics covered in this show:
- Walunas became interested in computer science during her postdoctoral work studying immunology and she obtained a master’s degree in computer science after completing her PhD. This led to her current interest using data from electronic medical records (EMR) in her research.
- Using big data from EMRs to measure quality of care is one of the projects Walunas has worked on, but she is also excited about using other data from clinical care to help model human disease.
- A lot of the current research using EMRs has been conducted at well-resourced academic medical centers, Walunas says, where teams using EMRs know how to record patient data well. She is working on projects that help to train clinicians and staff in smaller clinics to record data in the EMR and work on interventions that will lead to better outcomes for their patients.
- Walunas was part of a team that recruited 226 small practices across the Midwest using 15 practice coaches/facilitators to help practitioners deploy evidence-based clinical care and engage with the EMR and maintain this practice She says practices that engaged in more coaching-based strategies with practice facilitators are more likely to implement more quality improvement interventions.
- Walunas also conducts research on improving health outcomes for people with autoimmune diseases, particularly lupus. She realized that people often get sick because they don’t get the care they need, partly because they aren’t identified as needing that kind of care early enough. As a result, Walunas is using tools like machine learning to identify these patients before they get sick and potentially improve their overall outcomes.
- Immunotherapy cancer treatment can cause unintended adverse effects in certain patients, which present a lot like autoimmune disease. Walunas is using big data to try and understand which patients are more likely to get sick from immunotherapy. She hopes this type of research and understanding can help inform patients with primary autoimmune diseases as well. This research requires a varied team of immunologists, oncologists, machine learning experts, medical informatics and patients to be successful.
- The Institute for Artificial Intelligence in Medicine at Feinberg (I.AIM) offers a space to conduct computational phenotyping, and a “data gymnasium” where people can come and work with large and complicated datasets to understand how they behave and develop strategies for working with them. Walunas works closely with I.AIM director Abel Kho, MD on many of her projects.
- She says some skeptics may think that EMR data is “tainted” because it’s tied to the medical billing system, but Walunas explains that this EMR data coming from institutions such as Northwestern Medicine is a huge treasure trove of valuable information. Others may believe the EMR data and machine learning will fix everything, but Walunas explains that this is just another tool to help scientists augment their work and find new ways to improve patient care.
Additional Reading
- Read the her study on effective quality improvement strategies for small practices published in Medical Care
- “Evaluation of structured data from electronic health records to identify clinical classification criteria attributes for systemic lupus erythematosus” published in Lupus Science Medicine
- “Development of preclinical and clinical models for immune-related adverse events following checkpoint immunotherapy: a perspective from SITC and AACR” published in The Journal for Immunotherapy Cancer
Listen and Subscribe Here:
Erin Spain, MS [00:00:10] This is Breakthroughs, a podcast from Northwestern University Feinberg School of Medicine. I'm Erin Spain, host of the show. Big data is being used in transformative ways in healthcare and medical research. Today's guest, Theresa Walunas, is an informaticist and an immunologist who is using big data and implementation science at Northwestern Medicine and beyond to improve clinical care. She joins me today to talk about her research and share insights on this growing field. Dr. Walunas is an assistant professor of medicine in the Division of General Internal Medicine. She is also the associate director of CHIP, the Center for Health Information Partnerships. Welcome to the show.
Theresa Walunas, PhD [00:00:54] Oh, thank you. Thank you for having me. I am excited to be here today.
Erin Spain, MS [00:00:57] Well, I want to talk about your background. You're an informaticist and an immunologist. Tell me, what led you into these two very different fields and the work that you're doing here at Feinberg?
Theresa Walunas, PhD [00:01:07] Well, I started off my career as a as a bench trained immunologist in the nineties. So I say that because this is sort of before the human genome, before a lot of the big data that we think about was in place. And I was the immunologist in the lab who had figured out the email system. So by definition, that made me the computer scientist. And I started to get really excited about thinking about what would happen if we took this stuff that we're doing here at the bench in small scale. What would happen if we had all this larger scale information that wasn't just about mice, but was also about humans? Right. Because at the time we were very focused on mouse models because getting large numbers of humans to do any kind of study was tough. So after the end of my postdoc, I thought it would be a great idea to get a master's degree in computer science. That was a lot of fun and I learned a lot. And I spent the following ten years in a microbial genomics company actually developing my bioinformatics skills. But the thing about that was, is that it just it always seemed like we were so missing something. We were studying the microbes, but we were not studying the people quite as much. And in 2010, I actually had the opportunity to come back to Northwestern and work with a faculty member here who's the director of CHIP, Abel Kho, who is trying to figure out like how we could get more electronic health records into the hands of clinicians, because this was actually at the beginning of era high tech, the American Recovery and Reinvestment Act of 2009. And one of the big pieces of it was trying to get medical records adopted across the United States because, believe it or not, as of 2010, very, very few health care organizations consistently documented anyone's health care in the electronic health records. So in 2010, I came back to Northwestern and, you know, it wasn't a far leap, right, to think like, wow, if we're getting all this data in a medical record, maybe we can start to think about how to build human models. And so that's really the first piece of it. And the second part of what I got excited about was, well, while it's great that you have all this data, but have to be honest about medical records, doctors hate them, patients don't like them because they get between them and their doctor and they take up an incredible amount of time and they're primarily billing system. And if we want to use them for research, I think we need to do a couple of things. One is, is that we need to make sure that good data is going in. But in order to make sure good data is going in, I think we have to make sure that the physicians and the health care staff are getting something back from them. Right. Because ideally they should be one of these tools, I think, that physicians want to document. Well, that the whole medical team wants to document well in them because they're going to derive insights from that data that will help them provide better clinical care. Because if I learned anything from working with primary care people, it's that they really just want to provide good care. That's also where the implementation science comes in. It's like, okay, we have to think about sort of how we change patterns so that folks can document well, they can use things effectively and they can figure out how to translate what they're learning in addition to all the incredible clinical knowledge they have back into the clinic.
Erin Spain, MS [00:03:55] From 2010 to now 2022, a big transformation has taken place. Give me an idea of what's happening at Northwestern and the U.S. around the world with electronic medical records. What are we seeing as far as medical research and ways that people are able to improve quality of care?
Theresa Walunas, PhD [00:04:12] Well, in 2010, again, a very small percentage of the health care organizations were using electronic health records. As of 2015, roughly 95% of hospitals that take Medicare, which is most hospitals and about 80% of ambulatory care sites, were up on electronic health records. Now, that's, I think, exciting. But also, like any technology, it needed some additional support. So the scope is quite broad and you can imagine an amazing number of things that you could do as long as you can trust the data. And I think that's still even now in 2022, I think that's a big area of inquiry for the field is how do we make sure we can trust this data? How do we learn to validate it, what parts of it are good and how can we use it effectively? And you can use it in a lot of ways. Obviously the most basic ways are things like quality of care. In other words, you know, if you can track what care is being delivered to a patient, you can assess whether they're getting the things they're supposed to or not. You can assess whether their particular chronic conditions are managed. There's all sorts of information in the back end that can tell you how fast and how much. And so, again, that's sort of how we can think about improving quality of care. But the piece I get really excited about, too, is the modeling of people. One of the neat things about clinical data is that it is a record of people's clinical care, and it includes their labs, it includes their medications, it includes all sorts of information about their particular diagnoses and their particular conditions. It's all sorts of demographics and vital science. We've just not had that before to think about how we can model human disease. And so we still have a long way to go. But I think it's a fascinating place to start from the perspective of really thinking about patient-centered research, because we have that data already and we then don't necessarily have to go back and re collected from the patient either. So in ideal world, we can actually reduce the burden on patients and clinicians and then that doesn't even get at the whole issue of thinking about like population health and using data from a place like Northwestern intersected with multiple other organizations in the city. And there's a network here, the Chicago Area Patient-Centered Outcomes Research Network that just this that integrates data from health care organizations across the city. And when we use data in that context, we can actually try to understand better the sort of overall health of our community using clinical data. So I think there's a wide quality of care, clinical research and medical research and mechanistic research. And then actually we can also think about population health and public health strategies.
Erin Spain, MS [00:06:33] Tell me about some of the projects that you've worked on and that you are working on that involve these smaller clinics that you're trying to train physicians and people working in these clinics to use patient data to take good data and use the electronic medical record. Tell me about some of these projects and the aims of them.
Theresa Walunas, PhD [00:06:51] Maybe a good starting point is to say that a lot of our clinical trials in a lot of the places where we developed evidence are larger academic medical centers. These are awesome places, right? Because that's what they're there for. They are research hubs, but they're also usually really well staffed. The clinicians and people who are in this let's understand research and why we're doing it. They're able to maybe take some time and perform an experiment in a more systematic way. One of the things that's really challenging is a lot of these so-called, you know, we develop the evidence for high quality care, but we have to translate it into other environments, right. It's not enough just to sort of support our academic medical centers. We have to think about like, how is it that all clinicians across the country, no matter where they practice, are able to use these evidence-based practices to support their patients? And so one thing that it's a focus of implementation science, but is also something I think we can think how is it that we translate that? So the Agency for Health Care Research and Quality ARC has funded their Evidence Now program, and that's really about taking, you know, sort of evidence based practice and finding out how that evidence based practice works in other kinds of settings and what are the strategies we can use to implement that kind of work in in community settings. And so Dr. Kho and I actually we were part of the first phase of Evidence Now which was focused on trying to figure out how we could improve cardiovascular quality in primary care settings. As part of that, we recruited 226 small practices across Illinois, Indiana and Wisconsin. We deployed a force of about 15 practice coaches, and these practice coaches went into the clinics with a set of interventions to improve the so-called ABCs of cardiovascular health. Asprin for an ischmic vascular disease, blood pressure management, cholesterol management and smoking cessation. And our practice coaches essentially worked with each practice to assess where they were, what they could do, what they wanted to do, and then help them sort of implement a series of interventions that would allow them to improve health around these measures in sort of an electronic health record focused way. And the coach was there for the whole time to guide them and to see if they could help them get it done and also hopefully help them sustain what they learned, because that's the important part, too. It's not good enough for us to deploy something, have people leave, and then have the practice not be able to do it. They needed to be able to maintain it. And that's actually what we learned in age three, was that with the support of practice coaching, our small practices could implement and sustain change for things like blood pressure management, smoking cessation, so forth.
Erin Spain, MS [00:09:16] And you published about this?
Theresa Walunas, PhD [00:09:17] Well, we have two publications. So one is the primary results. I mean, that was in Medical Care and that was essentially looking at sort of the overall study. What I'm very interested in is once we know what works, how is it that we effectively and consistently can go into new settings and make that intervention work for other people? And so what I wanted to do was understand, well, why did practice facilitation work? And a lot of people have argued, but it's not been well studied. Well, it's the coaching, of course. It's the coaching that matters. But they do a lot of things. Practice facilitators, actually. You know, there's a spectrum of things that they do all the way from the very sort of simple, you might imagine, like tasks that they might do for the practice, right? They might call up an electronic health record vendor, they might set up a meeting or they. Might do things that sort of are very visceral activities that the practice needs done. Then you can manage on the other end of the spectrum that they're functioning like a coach. They're not really doing much for the practice at all, but maybe they're helping convene and maybe they're helping guide and maybe it's like, you know, it's like your personal trainer at the gym, right? They're not lifting for you, but they're helping you figure out how you can continue to lift weights so that you can get better and stronger what you want to do. That's what we postulated our practice facilitators might be doing for their practices. So we looked at the things the facilitators were doing. We group them, and then we essentially did this nice little machine learning study to try to understand what things the facilitators did that the coaches did were associated with success in implementing the number of interventions. And what we found was actually coaching matters was that compared to just doing task focused work, the facilitators who were able to deliver more coaching and sort of more interventions that helped the practice but didn't necessarily do for them. The more successful the practices were in the interventions that they implemented.
Erin Spain, MS [00:10:58] You also have a specific research focus on improving health for people with autoimmune diseases. Tell me how you use genomics informatics and implementation science methods to do that.
Theresa Walunas, PhD [00:11:09] One of the things that I think sort of changed my world was when I got here and I started to think differently about immune disease. And part of that was my work with the rheumatologist here, Dr. Rosalind Ramsey Goldman. And she's very focused on population health for people with lupus. When I was at the bench, I spent a lot of time thinking, well, if we can just give people this drug, it will change everything, right? If we can understand how their immune system works, we can fix it. And working with her was really eye opening because it also made me realize that part of the reason people can get so sick sometimes is simply because they don't get the care they need. They don't get access to care. We don't identify them again, as as a bench person. I never really occurred to me to think that way. And so that's sort of really where we start to put it all together is like, well, we also, in addition to knowing how to provide people medications and good therapy when they get sick, we also need to think about how do we find them early so that they can actually get the care they need? How do we make open up more gateways to care? And then once they get into the care system, how do we make sure they're getting good preventative screenings? How do we make sure that we're using their information effectively to get them the best possible care for their particular condition? How can we understand the specifics of them, the so called precision medicine right to sort of target the right thing to the right patient at the right time? And so that's kind of how this all came together is like I feel like I'm this really exciting place working with the kind of big data that we have here at Northwestern and through Capricorn, where we can start to really understand all the pieces of it, right? We can understand what's going out in the population. We can use machine learning and some of these strategies to identify different populations of patients and try to understand what's going on with them, what they might need differently. And then when we get into care, hopefully we can also use this information to help clinicians do really patient specific things in the setting, whether they mean more preventive screenings, whether they need particular medications, whether they need access to particular therapeutic regimens. You know, that's I live in the dream. I think we're really trying to find sort of a soup to nuts strategies where we can start at the population level and work to the individual level. In terms of how we think about improving health outcomes from immune disease.
Erin Spain, MS [00:13:13] You've published about the implementation, you've published about using electronic medical records for this kind of modeling of human diseases. You recently published a review focused on building infrastructure to support cancer immunotherapy, adverse events, research. Tell me about that.
Theresa Walunas, PhD [00:13:29] Well, interestingly enough, there's a whole new class of drugs for cancer, and they're drugs that are based on modulating the immune system behavior. And in 2018, they won the Nobel Prize. I mean, they've revolutionized care for many cancers. Unfortunately, when you manipulate the immune system, you sometimes manipulate it in ways that have events occur that you don't like very much, and that's these so-called immune related adverse events. What's interesting is that some of these look like autoimmune disease. One of the big challenges with autoimmune disease and cancer is it's really hard to model certain pieces of them in animals. And so some studies we can absolutely do in animals, but the adverse events, it's not one of those things that we've found good sort of so-called preclinical or animal models. And so this is a place where the human data shines in that people who get cancer and get an immunotherapeutic, they hit the health care system a lot. Right? So we have a lot of their data. They also get a lot of tests. So we understand a lot of them from a laboratory perspective. Many of them are part of trials, genomic studies. So we're starting to build really large bodies of data. And then, of course, there's also a lot of these so-called immuno toxicity services where there are groups of clinical fellows who focus on particular specialties but also, you know, can gather tissues. Is a community now that's trying to figure out how can we use all the data that we have to better understand who's going to develop these adverse events, how to care for them. So some of them can be very severe. So how do we make sure that patients have the best possible outcomes? And of course, because some of these look like autoimmune diseases, my hope is that we might be able to study them understand whether it can help people with primary autoimmune diseases as well. What's exciting about that paper is it was a large group of researchers that came together that was supported by the Society for Immunotherapy for Cancer, as well as the American Association of Cancer Research. We came out of that room going, Well, what is it that we really need to do to take the next step? And one hand, we need a lot of great clinical data that we can use to build models of people that kind of lives at the center. We need to find ways to intersect data, both primary mechanistic data, as well as environmental and social determinants data to help better understand our patients. And that's just the data. How do you interpret it? You need to build a body of tools to help you validate the data, understand what's good data, what's bad data, what's missing, and how you fix it. You need tools to find different types of people with different types of things going on with them in the data. You need a whole collection of fancy machine learning strategies to sort of figure out how you can identify people and how okay, if this person has this particular adverse event, what are some of the mechanistic and environmental and social determinants types of attributes that might be associated with that adverse event? Can we use that to change care? And last but not least, and I think the really important is we need a really diverse, multidimensional community of people who can support this work. So we need immunologists, we need oncologists, we need machine learning people, we need medical informatics, we need patients. We need all sorts of different types of folks who have different areas of expertise to kind of come together, help us interpret the data, help us figure out what questions to ask, how to get different type of tissue samples. I mean, it's a very, very complicated space. And so I think that's what that paper hoped to sort of set out is like a kind of like a manifesto for sort of the next generation of research into this space.
Erin Spain, MS [00:16:50] Earlier, you mentioned Dr. Abel Kho, who's the director of the Institute for Augmented Intelligence in Medicine [now called the Institute for Artificial Intelligence in Medicine] at Feinberg. Tell me, what are some of the things that are happening at Northwestern Medicine and Feinberg that you think are really supporting this next big step that's needed?
Theresa Walunas, PhD [00:17:06] I.AIM, the Institute for Augmented Intelligence in Medicine [now called the Institute for Artificial Intelligence in Medicine] is definitely one of those places where things are starting. It's got a really exciting center for computational phenotyping, which basically means finding cohorts of people in complicated datasets. He's also got a really incredible set of goals to kind of try to bring together more datasets and create these kinds of the data gymnasium where people can come and find data and mess around with it and learn how to use it and develop strategies for working with it. So, I think that's one piece. I think our informatics community in general is really trying to find more ways to get together, meet each other, figure out what's going on and how to use it. There are a wide variety of quality improvement programs that are going on here at Northwestern that are trying to use machine learning and augmented sort of intelligence strategies to figure out how we develop clinical decision support for people with congestive heart failure. One of my colleagues Faraz Ahmad is working in that space with his K. Dr. Ramsey Goldman and I are working in terms of lupus at trying to figure out whether we can find people with lupus based on the way their disease presents as opposed to the way they're diagnosed, so that you can kind of identify preventive care strategies for them. It's a growing community, and that doesn't even begin to kind of get into how people are focused in the implementation science space to think about how do we take this data and sort of translate it into interventions that we can then use to improve care with.
Erin Spain, MS [00:18:28] What are some common misconceptions that you encounter about the work that you do, the role of big data in clinical care?
Theresa Walunas, PhD [00:18:36] Oh, it's funny. There's two ends of the spectrum. There's a group that's like basically everybody thinks this data is horrible, medical record data is new and it comes out of a billing system. Right. And we always have to be skeptical a little bit about how we use it, why we use it and what it can mean. And it can be subject to a lot of different types of interpretation. But I think actually the thing we need to remember is who's putting that data into the system? Well, it's the amazing team of people here at Northwestern Medicine, right, with the clinical training and expertise to take care of patients. When we look at it that way, it's just an incredibly rich treasure trove. So I always try to dissuade people from being so negative about it because I think it really is in many ways an expert curated data source. On the other end of the spectrum, there's the people who believe it will do everything. And I think that it's not magical and machine learning is not magical. And we still need to figure out how to make sure we have good models, how to understand those models, to sort of test those models in a variety of way before we put them into practice and to make sure we can figure out how to explain them. Right. Because when you're sitting with a patient, I can only imagine saying, well, the computer said this is what we should do, isn't going to be very satisfying. So we also have to think about how we use machine learning. And that's why the institute has the name augmented intelligence, because what we're trying to do is not have the machine think for us, but trying to have the machine help us weed through the data and identify the things that are important so that our clinical care team members can make really good decisions about how to improve care and make sure patients at Northwestern Medicine get the best care possible.
Erin Spain, MS [00:20:01] Well, thank you so much, Dr. Theresa Walunas, for coming on the show, talking about your work and so many exciting projects that are happening in Northwestern Medicine and Feinberg, we really appreciate it.
Theresa Walunas, PhD [00:20:12] Thanks for having me.
Erin Spain, MS [00:20:21] And thanks for listening. And be sure to subscribe to this show on Apple Podcasts or wherever you listen to podcasts and rate and reviews. Also for medical professionals, this episode of Breakthroughs is available for CME Credit. Go to our website, Feinberg.Northwestern.edu and search CME.
Physicians who listen to this podcast may claim continuing medical education credit after listening to an episode of this program.
Target Audience
Academic/Research, Multiple specialties
Learning Objectives
At the conclusion of this activity, participants will be able to:
- Identify the research interests and initiatives of Feinberg faculty.
- Discuss new updates in clinical and translational research.
Accreditation Statement
The Northwestern University Feinberg School of Medicine is accredited by the Accreditation Council for Continuing Medical Education (ACCME) to provide continuing medical education for physicians.
Credit Designation Statement
The Northwestern University Feinberg School of Medicine designates this Enduring Material for a maximum of 0.25 AMA PRA Category 1 Credit(s)™. Physicians should claim only the credit commensurate with the extent of their participation in the activity.
Disclosure Statement
Theresa Walunas, PhD, has received grant or research support from Gilead Sciences, Inc. Content reviewer Gigi Dawood, DO, has nothing to disclose. Course director, Robert Rosa, MD, has nothing to disclose. Planning committee member, Erin Spain, has nothing to disclose. Feinberg School of Medicine's CME Leadership and Staff have nothing to disclose: Clara J. Schroedl, MD, Medical Director of CME, Sheryl Corey, Manager of CME, Allison McCollum, Senior Program Coordinator, Katie Daley, Senior Program Coordinator, Michael John Rooney, Senior RSS Coordinator, and Rhea Alexis Banks, Administrative Assistant 2. All the relevant financial relationships for these individuals have been mitigated.